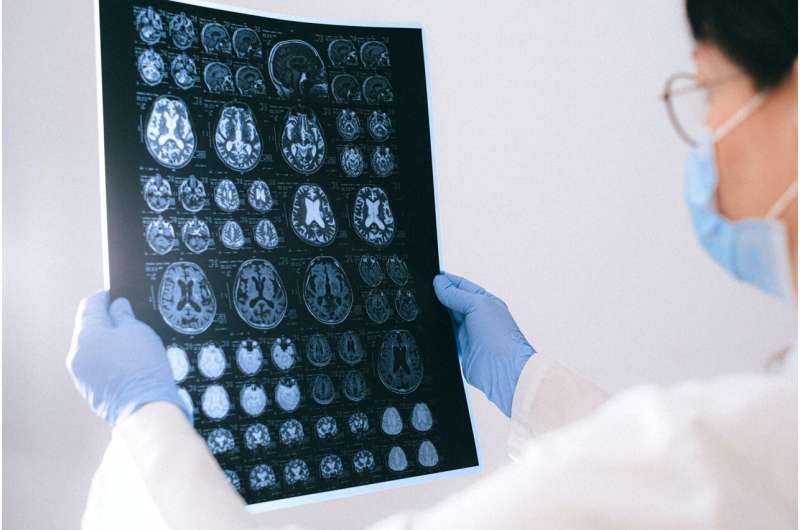
A paper titled “Deep Learning and Transfer Learning for Brain Tumor Detection and Classification” published in Biology Methods and Protocols shows that scientists can train artificial intelligence (AI) models to distinguish brain tumors from healthy tissue. AI models can already find brain tumors in MRI images almost as well as a human radiologist.
Researchers have made sustained progress in AI for use in medicine. AI is particularly promising in radiology, where waiting for technicians to process medical images can delay patient treatment. Convolutional neural networks are powerful tools that allow researchers to train AI models on large image datasets to recognize and classify images.
In this way, the networks can “learn” to distinguish between pictures. The networks also have the capacity for “transfer learning.” Scientists can reuse a model trained on one task for a new, related project.
Although detecting camouflaged animals and classifying brain tumors involves very different sorts of images, the researchers involved in this study believed that there was a parallel between an animal hiding through natural camouflage and a group of cancerous cells blending in with the surrounding healthy tissue.
The learned process of generalization—the grouping of different things under the same object identity—is essential to understanding how a network can detect camouflaged objects. Such training could be particularly useful for detecting tumors.
In this retrospective study of public domain MRI data, the researchers investigated how neural network models can be trained on brain cancer imaging data while introducing a unique camouflage animal detection transfer learning step to improve the networks’ tumor detection skills.
Using MRIs from public online repositories of cancerous and healthy control brains (from sources including Kaggle, the Cancer Imaging Archive of NIH National Cancer Institute, and VA Boston Healthcare System), the researchers trained the networks to distinguish healthy vs. cancerous MRIs, the area affected by cancer, and the cancer appearance prototype (what type of cancer it looks like).
The researchers found that the networks were almost perfect at detecting normal brain images, with only one to two false negatives, and distinguishing between cancerous and healthy brains. The first network had an average accuracy of 85.99% at detecting brain cancer, the other had an accuracy rate of 83.85%.
A key feature of the network is the multitude of ways in which its decisions can be explained, allowing for increased trust in the models from medical professionals and patients alike. Deep models often lack transparency, and as the field grows, the ability to explain how networks perform their decisions becomes important.
Following this research, the network can generate images that show specific areas in its tumor-positive or negative classification. This would allow radiologists to cross-validate their own decisions with those of the network and add confidence, almost like a second robotic radiologist who can show the telltale area of an MRI that indicates a tumor.
In the future, the researchers here believe it will be important to focus on creating deep network models whose decisions can be described in intuitive ways, so artificial intelligence can occupy a transparent supporting role in clinical environments.
While the networks struggled more to distinguish between types of brain cancer in all cases, it was still clear they had distinct internal representation in the network. The accuracy and clarity improved as the researchers trained the networks in camouflage detection. Transfer learning led to an increase in accuracy for the networks.
While the best performing proposed model was about 6% less accurate than standard human detection, the research successfully demonstrates the quantitative improvement brought on by this training paradigm. The researchers here believe that this paradigm, combined with the comprehensive application of explainability methods, promotes necessary transparency in future clinical AI research.
“Advances in AI permit more accurate detection and recognition of patterns,” said the paper’s lead author, Arash Yazdanbakhsh.
“This consequently allows for better imaging-based diagnosis aid and screening, but also necessitates more explanation for how AI accomplishes the task. Aiming for AI explainability enhances communication between humans and AI in general. This is particularly important between medical professionals and AI designed for medical purposes.
“Clear and explainable models are better positioned to assist diagnosis, track disease progression, and monitor treatment.”
More information:
Faris Rustom et al, Deep Learning and Transfer Learning for Brain Tumor Detection and Classification, Biology Methods and Protocols (2024). DOI: 10.1093/biomethods/bpae080
Citation:
Radiologists could soon be using AI to detect brain tumors (2024, November 19)
retrieved 19 November 2024
from
This document is subject to copyright. Apart from any fair dealing for the purpose of private study or research, no
part may be reproduced without the written permission. The content is provided for information purposes only.
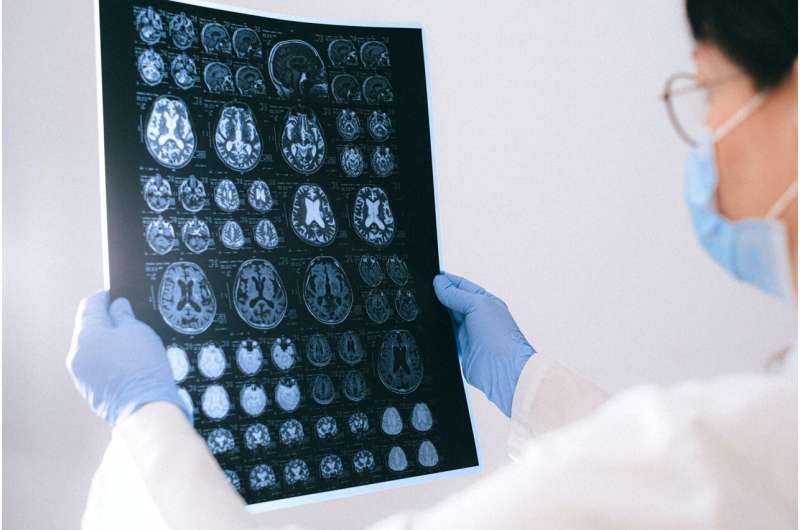
A paper titled “Deep Learning and Transfer Learning for Brain Tumor Detection and Classification” published in Biology Methods and Protocols shows that scientists can train artificial intelligence (AI) models to distinguish brain tumors from healthy tissue. AI models can already find brain tumors in MRI images almost as well as a human radiologist.
Researchers have made sustained progress in AI for use in medicine. AI is particularly promising in radiology, where waiting for technicians to process medical images can delay patient treatment. Convolutional neural networks are powerful tools that allow researchers to train AI models on large image datasets to recognize and classify images.
In this way, the networks can “learn” to distinguish between pictures. The networks also have the capacity for “transfer learning.” Scientists can reuse a model trained on one task for a new, related project.
Although detecting camouflaged animals and classifying brain tumors involves very different sorts of images, the researchers involved in this study believed that there was a parallel between an animal hiding through natural camouflage and a group of cancerous cells blending in with the surrounding healthy tissue.
The learned process of generalization—the grouping of different things under the same object identity—is essential to understanding how a network can detect camouflaged objects. Such training could be particularly useful for detecting tumors.
In this retrospective study of public domain MRI data, the researchers investigated how neural network models can be trained on brain cancer imaging data while introducing a unique camouflage animal detection transfer learning step to improve the networks’ tumor detection skills.
Using MRIs from public online repositories of cancerous and healthy control brains (from sources including Kaggle, the Cancer Imaging Archive of NIH National Cancer Institute, and VA Boston Healthcare System), the researchers trained the networks to distinguish healthy vs. cancerous MRIs, the area affected by cancer, and the cancer appearance prototype (what type of cancer it looks like).
The researchers found that the networks were almost perfect at detecting normal brain images, with only one to two false negatives, and distinguishing between cancerous and healthy brains. The first network had an average accuracy of 85.99% at detecting brain cancer, the other had an accuracy rate of 83.85%.
A key feature of the network is the multitude of ways in which its decisions can be explained, allowing for increased trust in the models from medical professionals and patients alike. Deep models often lack transparency, and as the field grows, the ability to explain how networks perform their decisions becomes important.
Following this research, the network can generate images that show specific areas in its tumor-positive or negative classification. This would allow radiologists to cross-validate their own decisions with those of the network and add confidence, almost like a second robotic radiologist who can show the telltale area of an MRI that indicates a tumor.
In the future, the researchers here believe it will be important to focus on creating deep network models whose decisions can be described in intuitive ways, so artificial intelligence can occupy a transparent supporting role in clinical environments.
While the networks struggled more to distinguish between types of brain cancer in all cases, it was still clear they had distinct internal representation in the network. The accuracy and clarity improved as the researchers trained the networks in camouflage detection. Transfer learning led to an increase in accuracy for the networks.
While the best performing proposed model was about 6% less accurate than standard human detection, the research successfully demonstrates the quantitative improvement brought on by this training paradigm. The researchers here believe that this paradigm, combined with the comprehensive application of explainability methods, promotes necessary transparency in future clinical AI research.
“Advances in AI permit more accurate detection and recognition of patterns,” said the paper’s lead author, Arash Yazdanbakhsh.
“This consequently allows for better imaging-based diagnosis aid and screening, but also necessitates more explanation for how AI accomplishes the task. Aiming for AI explainability enhances communication between humans and AI in general. This is particularly important between medical professionals and AI designed for medical purposes.
“Clear and explainable models are better positioned to assist diagnosis, track disease progression, and monitor treatment.”
More information:
Faris Rustom et al, Deep Learning and Transfer Learning for Brain Tumor Detection and Classification, Biology Methods and Protocols (2024). DOI: 10.1093/biomethods/bpae080
Citation:
Radiologists could soon be using AI to detect brain tumors (2024, November 19)
retrieved 19 November 2024
from
This document is subject to copyright. Apart from any fair dealing for the purpose of private study or research, no
part may be reproduced without the written permission. The content is provided for information purposes only.